Learn from what others have done
It all starts with individual condition monitoring of your every unit out there. You can predict future values based on what is normal, which will tell before a critical threshold is exceeded, or if there is a deviation between the expected (predicted) and the actual value. This empowers and enables a catalog of use cases for maintenance and performance optimization.
No unscheduled downtime. This is the objective of every industrial process. This requires that you constantly monitor the condition of the specific installation, in real-time, to detect deviations and ways to run better – something that machines are really good at doing, in particular with machine learning.
This resonates with minimizing human time spent on low-value routine work since fewer must do more, ensuring that these resources can focus their effort where needed, and getting relevant and coherent insights to solve problems and minimize human errors.
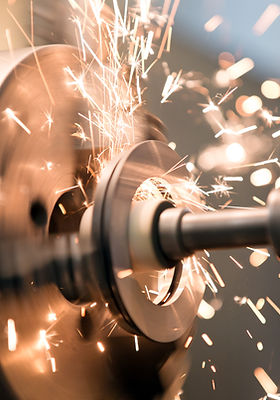
The use cases below demonstrate how industrial product OEMs use Ekkono’s Edge AI to calculate remaining time to service, remaining useful life (RUL) on wear parts, maximizing the utilization of wear parts, extending product life by avoiding fatal wear from break-downs, and to not only reduce unscheduled but also scheduled downtime by doing it when needed.
While every unit you produce might be equal, every installation is unique, and the customer expects to be treated accordingly. This individual, real-time, proactive approach to adaptive automation will meet all these requirements, but it will also empower you to do the service transformation that presents entirely new business models and opportunities.
Use Cases
Condition-Based Maintenance on Heat Exchangers
Heat exchangers are found everywhere in the process industry and manufacturing. Inefficiently run heat exchangers account for 2.5% of the global CO2 footprint. The issue is that the same type of heat exchanger can have between six weeks and five years of maintenance cycle depending on where it runs and what it heats/cools. Predicting when the efficiency will go beneath a critical level enables schedule cleaning when it is needed – proactive condition-based maintenance. By using temp and pressure, Ekkono’s Edge AI creates a model that predicts remaining time to service. The savings are big based on servicing only when needed, minimizing production stops, but in time to avoid that it runs inefficiently.

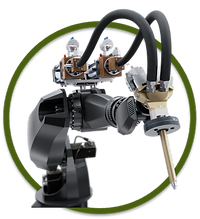
Detect Nozzle Clogging on Adhesive Robot
Atlas Copco provides adhesive robots that mount windshields to car OEMs. Every car factory is unique and they use different glues. But more importantly, the car OEMs won’t let Atlas Copco extract any data from the premises, which means that all intelligence must reside on the robot controller. Said and done, resulting in fewer unscheduled stops due to clogged nozzles by detecting it proactively, changing the nozzle temperature, and doing this without having a service engineer going onsite. Read more in this article.
Gas Burner Tuning on Gas Turbines
Gas turbines operate in different conditions where the oxygen mix depends on altitude, temp, and humidity, meaning location and season. To run at its best each of the 8-30 gas burners must be individually tuned to create the perfect flame. Automating this using AI, keeping them constantly up to date instead of manually tuning them once per year, reduces NOx emissions by over 4%; While also saving on travel for the turbine experts who do not have to go on site.

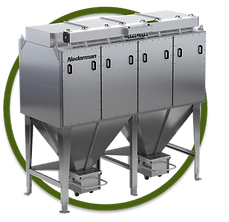
Filter Monitoring
Filters are central to the performance of ventilation solutions. In particular for industrial air solutions that can exhaust saw dust or welding fumes. Learning and predicting the pressure over the filter determines the performance and if automated cleaning or filter replacement is required. The “right” pressure depends on what is filtered, unit configuration, and pipe dimension/length. Learning the individual conditions improves the precision when monitoring the filter performance, enabling automated cleaning, remote maintenance, filter subscriptions, SLAs (service level agreements), and even added value services.
Detect Bad Sectors on Saw Blade
Stone saws are power tools that require high safety. Detecting bad sectors (missing diamonds) or glazing can’t be done with a sensor or camera on the rotating blade. But by looking at the power consumption (voltage and current) of the individual stone saw, Ekkono’s Edge AI is able to detect when these bad sectors start to emerge. This could not be done with a generic model for all stone saws, but works only when learning and detection is done on the individual unit and how it is used.


Remain Range on Electrical Buses
By adding surrounding conditions like climate, landscape, type of roads and traffic conditions, the precision of the remaining range calculation is significantly improved. And by learning this onboard the vehicle, Ekkono can automatically incorporate these conditions into the model. The added benefit of city buses that continuously operate the same route is that the prediction can be enhanced even further. This not only removes range anxiety but also enables better route and charge planning.
Pump-as-a-Sensor
Pumps are used for various applications like pumping beer at a brewery or pumping drain water at a farm. This means that what’s normal for one is an anomaly for another. Individual learning in operations is the only way to properly monitor the condition of the pump. But doing so will not only tell you the status of the pump but also if there’s an issue elsewhere, like a leaking pipe. This turns the pump into a smart and adaptive sensor. Imagine when not only the pump but also the compressor, fan and motor can tell the health of your process!
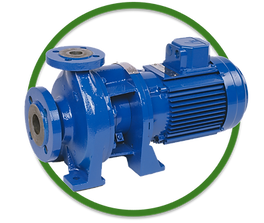
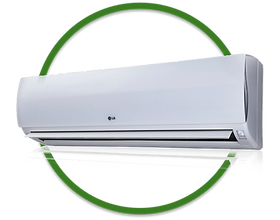
Self-Configuring Air Conditioners
There are 5.5 billion air conditioners in the world. Most of them run standard factory settings, independent if it runs in Gothenburg or Kuala Lumpur, if it’s summer or winter, if it’s in a hotel, office or residence, and whether people are in the room or not. Imagine an air conditioner that automatically learns the room, learning more and more over time, including daily, weekly and seasonal changes, to eventually predict when there will be people in the room or not and automatically control the temperature and thereby the power consumption accordingly. Yup, check on that!